Meta Launches Testing of Its First Proprietary AI Training Chip – One America News Network
By Katie Paul and Krystal Hu
March 11, 2025 – 6:37 AM PDT
Advertisement
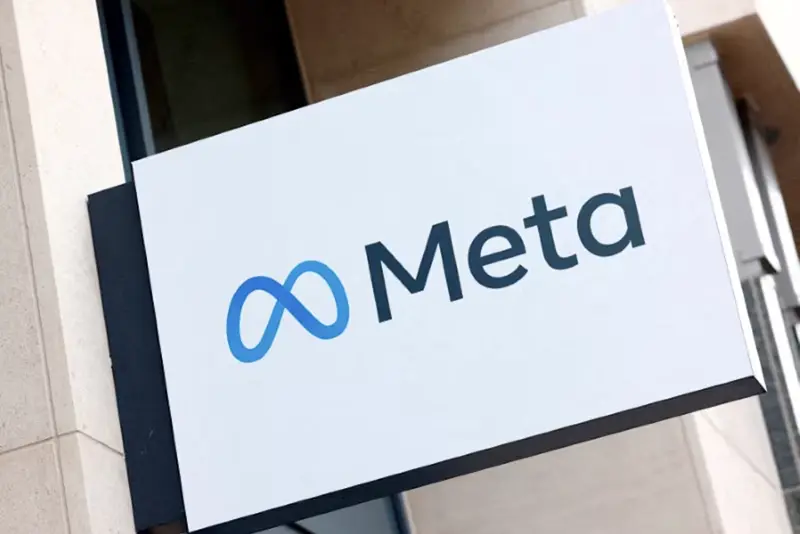
NEW YORK (Reuters) – Meta Platforms, the parent company of Facebook (META.O), is currently testing its inaugural in-house chip designed for training artificial intelligence systems. This marks a significant step towards creating more customized silicon and decreasing dependency on external providers, such as Nvidia (NVDA.O), according to two sources cited by Reuters.
The largest social media platform has initiated a limited deployment of the chip and aims to increase production for a broader application if the trials are successful, the sources noted.
This initiative to create proprietary chips is part of Meta’s long-term strategy to lower its enormous infrastructure expenses, as the company invests heavily in AI technologies to stimulate growth.
Meta, which also owns Instagram and WhatsApp, anticipates total expenses ranging from $114 billion to $119 billion for 2025, with capital expenditures reaching as high as $65 billion, largely due to investments in AI infrastructure.
According to one source, the new training chip from Meta is a specialized accelerator, tailored for handling AI-specific tasks. This design can enhance power efficiency compared to the traditional graphics processing units (GPUs) typically employed for AI operations.
Meta is collaborating with TSMC (2330.TW), a chip manufacturer based in Taiwan, to develop the chip, the source indicated.
The testing phase commenced following the successful completion of Meta’s inaugural “tape-out” of the chip, which is a critical achievement in the silicon development process that involves submitting an initial design to a chip fabrication facility, as per another source’s information.
Generally, a tape-out can cost millions of dollars and may take roughly three to six months to finalize, with no assurance of success. Should any issues arise, Meta would need to identify the problem and repeat the tape-out process.
Neither Meta nor TSMC provided comments on the matter.
This chip represents the latest addition to the Meta Training and Inference Accelerator (MTIA) series. The program has faced challenges over the years, even leading to the cancellation of a similar chip at a comparable stage of development.
Despite that, last year, Meta began utilizing an MTIA chip for inference tasks, which involves the operation of AI systems during user interactions, specifically for the recommendation algorithms that dictate content visibility on Facebook and Instagram’s news feeds.
Meta executives have expressed their intention to deploy their own chips for training purposes by 2026, focusing on the intensive computational task of educating the AI system with extensive datasets.
As with the inference chip, the planned application for the training chip will initially target recommendation systems, with future plans to extend its use to generative AI products like Meta AI chatbots, as indicated by company executives.
“We’re strategizing on how to implement training for recommendation systems and, over time, consider how to approach training and inference for generative AI,” stated Meta’s Chief Product Officer Chris Cox during the Morgan Stanley technology, media, and telecom conference last week.
Cox characterized Meta’s chip development journey as a “walk, crawl, run situation” so far, noting that executives view the first-generation inference chip used for recommendations as a substantial achievement.
Previously, Meta discontinued a custom in-house inference chip after it failed in a pilot test akin to the current deployment for the training chip, opting instead to place substantial orders for Nvidia GPUs amounting to billions in 2022.
Since then, the social media giant has remained one of Nvidia’s significant clients, building a collection of GPUs to train its models, including for recommendation systems, advertisements, and its Llama foundation model series. These units also handle inference tasks for the over 3 billion daily users of its applications.
The worth of these GPUs has come under scrutiny this year as AI researchers increasingly voice skepticism about the potential for further advancements by merely enhancing large language models through additional data and computing resources.
These concerns were amplified by the late-January introduction of cost-effective models from Chinese startup DeepSeek, which prioritize computational efficiency by focusing more on inference than traditional models.
Following a global downturn in AI stock prices triggered by DeepSeek’s announcement, Nvidia shares plummeted by as much as 20% at one point. Although they later regained most of their losses, investors seem to believe that Nvidia’s chips will continue to serve as the industry standard for training and inference, despite recovering recently under broader trade uncertainties.
Reporting by Katie Paul in New York and Krystal Hu in San Francisco; Editing by Richard Chang
Advertisements below